Agentic AI
Challenges of AI Integration
Overview of Agentic AI, a next-generation AI paradigm that moves beyond simple, reactive responses to autonomous problem-solving and proactive action. Unlike traditional AI systems or chatbots that primarily respond to explicit user prompts, Agentic AI systems can perceive their environment, reason about complex problems, plan solutions, act independently, and learn from their experiences. This technology has the potential to transform industries by enhancing productivity, personalizing customer experiences, streamlining workflows, and enabling new forms of automation.
Key Themes and Ideas
Definition and Core Functionality: Agentic AI is defined as AI systems that use sophisticated reasoning and iterative planning to autonomously solve complex, multi-step problems. It goes beyond the capabilities of traditional AI, such as chatbots that primarily respond to single-turn interactions. A defining characteristic is its ability to perceive, reason, act, and learn, creating a "data flywheel" for continuous improvement. This enables it to independently analyze challenges, develop strategies, and execute tasks. Distinction from Traditional AI/Chatbots: Traditional AI tools, like ChatGPT, function as "incredibly smart assistants" that wait for instructions and generate responses based on training data. Agentic AI is more like a "colleague" or "partner" that understands the bigger picture and can work independently towards goals. The analogy of waiters, moan and madav, illustrates the difference. moan is accurate but only follows directions, while madav is proactive, offering suggestions and anticipating customer needs, which represents Agentic AI.
How Agentic AI Works (Four-Step Process):
Perceive: Gather and process data from various sources (sensors, databases, digital interfaces). Reason: Use a large language model (LLM) as an orchestrator to understand tasks, generate solutions, and coordinate specialized models (e.g., content creation, visual processing).
Retrieval-Augmented Generation
(RAG) is used to access proprietary data. Act: Integrate with external tools and software via APIs to execute tasks based on formulated plans. Guardrails are implemented to ensure correct task execution. Learn: Continuously improve through a feedback loop, where data generated from interactions refines models and increases effectiveness.
Fueling Agentic AI with Data:
Agentic AI leverages vast amounts of data from diverse sources. Accelerated AI query engines and RAG techniques are crucial for efficient data processing, storage, and retrieval. The "data flywheel" enables continuous learning and improvement by feeding data generated through interactions back into the system. Applications Across Industries: Customer Service: Enhancing self-service capabilities, automating routine communications, and offering personalized support through AI-powered "digital humans." Quote: "Over half of service professionals report significant improvements in customer interactions, reducing response times and boosting satisfaction." Content Creation: Quickly creating personalized marketing content, saving marketers time and improving customer engagement. Quote: "Generative AI agents can save marketers an average of three hours per content piece, allowing them to focus on strategy and innovation." Software Engineering: Automating repetitive coding tasks to boost developer productivity and free them up for more complex challenges. Quote: "It’s projected that by 2030 AI could automate up to 30% of work hours, freeing developers to focus on more complex challenges and drive innovation." Healthcare: Distilling critical information for doctors to make better-informed care decisions, automating administrative tasks, and providing 24/7 patient support. **Video Analytics: Enable anomaly alerts, incident reports, and predictive maintenance through the analysis of video data Autonomy and Control
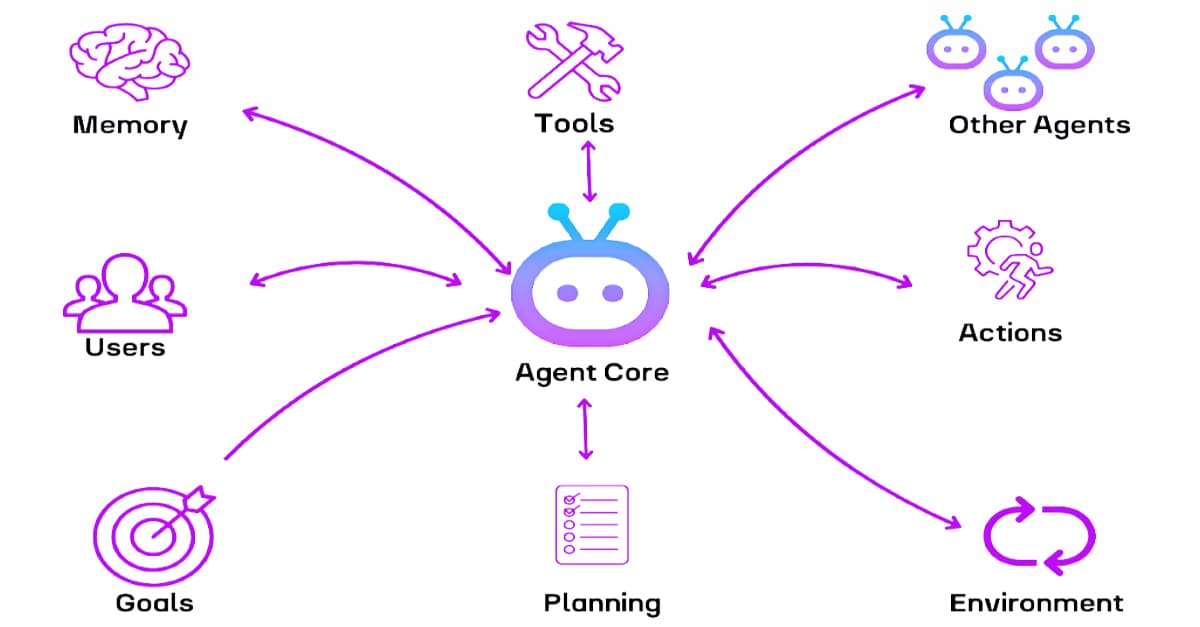
AI agents autonomy:
AI agents autonomy exhibit and independent thinking within definedlimitations. The analogy of a dog on a leash is used. The dog (AI agent) has freedom to roam within the leash's length (defined control parameters). While AI agents can make suggestions and decisions independently, there are controls and limitations implemented through code to prevent unwanted or harmful actions. Evolutionary Shift: Agentic AI represents a "transformation of AI from a sophisticated tool into something more like a partner in problem-solving and Innovation."
This shift could make today's advanced AI systems look basic in comparison. Tools and Frameworks: Frameworks like LangChain, Microsoft Autogen, and CrewAI are used to build AI agents. AI agents utilize tools like weather APIs, databases, and web search to gather information and make informed decisions. Potential Concerns:Ensuring alignment with human values and ethical considerations. Quote: "how do we ensure that these autonomous systems remain aligned with our human values how do we balance the benefit of automation with the need for human oversight these are crucial challenges we need to address" Balancing the benefits of automation with the need for human oversight.
- Data Preparation: Training AI models requires large amounts of high-quality data.
- Model Training and Tuning: Experimentation and tuning require ML expertise.
- Scalability: Ensuring AI-powered features can handle increased traffic is crucial.
- Cost: AI APIs and platforms can be expensive for large-scale applications.